AI-based monitoring of 3D printing
SWMS creates software for the additive manufacturing of large components. Printing is carried out using an industrial robot to which an effector is attached. During this printing process, however, there are always deviations, e.g. due to changes in the curing process or inaccuracies in the layer-by-layer application of the material. The project therefore aims to continuously monitor the entire 3D printing process using sensor components (such as nozzle and thermal cameras) in order to detect printing errors at an early stage.
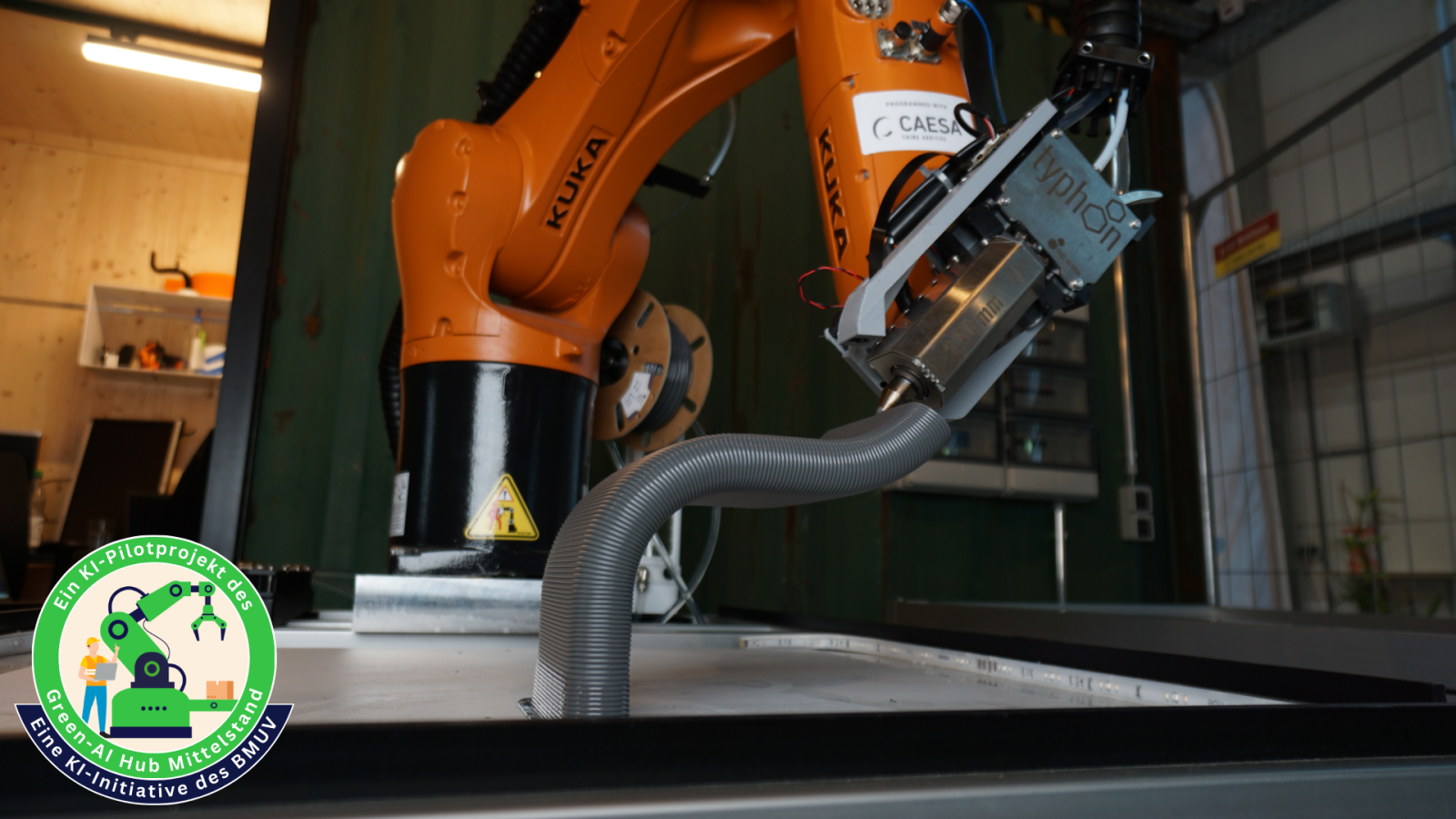
Software solutions for 3D printing made in Oldenburg
SWMS Systemtechnik Ingenieurgesellschaft mbH, founded in 1996, is a technology company for digital industrial solutions based in Oldenburg, Lower Saxony. With around 40 employees, the company offers software solutions in the complex field of automated composite manufacturing that support customers from the design of composite components through to their production. All activities are bundled in the CAESA software environment in corresponding modules and are available as system and manufacturer-independent programming and design software. Customers include companies from the aerospace, automotive, shipbuilding, and wind power industries.
Challenge: minimising printing errors through AI-supported monitoring
A major challenge for the company is to continuously monitor the 3D printing process to detect errors and subsequently cancel the printing process. Ideally, the print or component would be preserved through countermeasures such as active cooling. As additive manufacturing must also be economical for smaller batch sizes (up to batch size 1), the reliable production of components on the first attempt is necessary and essential.
When manufacturing composite components, flat components can be produced using traditional methods such as printing moulds. The stiffness of these components can be significantly increased by 3D printing support structures into this mould. However, if the 3D print fails, the entire component may be a reject.
Currently, quality control usually only takes place after the printing process has been completed. An ideal solution would be deviation detection and countermeasure implementation during the printing process. Simply recognising an error and stopping the printing process without taking countermeasures would already lead to material savings and an increase in resource efficiency.
AI-supported object recognition and image segmentation as a solution
This is precisely where artificial intelligence can come in. AI-supported object recognition or image segmentation can be used to identify printing errors and initiate countermeasures. The AI used here learns image-based object recognition and segmentation using deep learning approaches.
In order for the potential of the AI to be utilised, the AI model must first be trained accordingly. To do this, data from the sensors, such as the nozzle and thermal camera, must first be recorded and labelled manually. Training with labelled data is then necessary before the detection performance can be determined in use. Once the training is complete, live images from the camera can be monitored using the trained AI model, which can reduce or completely avoid production errors over time.
Value creation through AI for companies and customers
The component developed in the pilot project for error detection in 3D printing is to become part of a control software for non-planar 3D printing and is therefore an additional service component created for the customer. The component itself is used for continuous quality control of the production process. This results in several advantages, not only for the company itself but also for the customer.
Firstly, the aim is to integrate the AI component into the existing control software for additive manufacturing so that the component becomes part of a complete solution. Secondly, in the longer term, it should lead to a general improvement in the quality of additive 3D printing, as a broader basis can be created by continuously collecting sensor data to not only recognise errors, but also to determine and exclude the causes of errors.

Saving resources by integrating AI-supported monitoring into customer systems
In particular, the service and production areas of the value chain are addressed. SWMS is a technology company for digital industrial solutions and not a direct provider of 3D printing services, which means that the AI process will initially only have a minor impact (as part of testing and developing the pilot) on resource efficiency within the company. However, the integration of an error detection component into the control software for additive manufacturing will result in a multiplier effect, as the software can be used by many different companies.
The AI solution will have a significant impact on the consumption of the printing material, known as filament, as the printed objects will be continuously monitored for errors during the printing process; quality control will no longer take place only after the completion of the printing process. It is estimated that this can save around a third of the material used in desktop 3D printing. In addition, resources for operating and controlling the robot arm, heating the filament and, if necessary, actively cooling the print material are conserved.
The costs for the actual use of the AI solution are relatively low, as they are limited to the use of the trained AI model to analyse the visual data from the cameras, which can nowadays be carried out using standard hardware. Higher costs are incurred when training the AI models, as the training cycles generally require higher-performance hardware or access to computing clusters. In addition, costs arise from the manual labelling of the data and the use of sensors, computers to store the data, licence fees for the system software, and construction materials for the setup. How quickly the investment is amortised depends on several parameters, such as the print volume, the amount of filament used and the tolerance range for deviations in the print.
Presentation of the Green-AI Hub pilot project “AI-based monitoring of 3D printing“ - 16:47 min.
- Lars Windels, SWMS Systemtechnik Ingenieurgesellschaft mbH
- Nico Jordan, SWMS Systemtechnik Ingenieurgesellschaft mbH
- Christoph Tholen, German Research Center for Artificial Intelligence GmbH
Technology
AI capability: object recognition and image segmentation
AI model: computer vision
Value creation
Phase: production & service
Aim of AI: error detection and avoidance in 3D printing of components
Resource efficiency
GHG reduction potential of 2.53 tonnes CO2e
Savings in printing material (filament) and energy