AI-based quality assurance for rotogravure cylinders
4Packaging GmbH is a manufacturer of rotogravure cylinders, a critical component in packaging production that significantly affects the final product's appearance. To save material and energy in the production process, as well as to ensure high-quality standards, the company is implementing artificial intelligence (AI) for quality assurance and visual quality control. This involves retrofitting machines with additional sensors and analysing existing sensor data and images for each application.
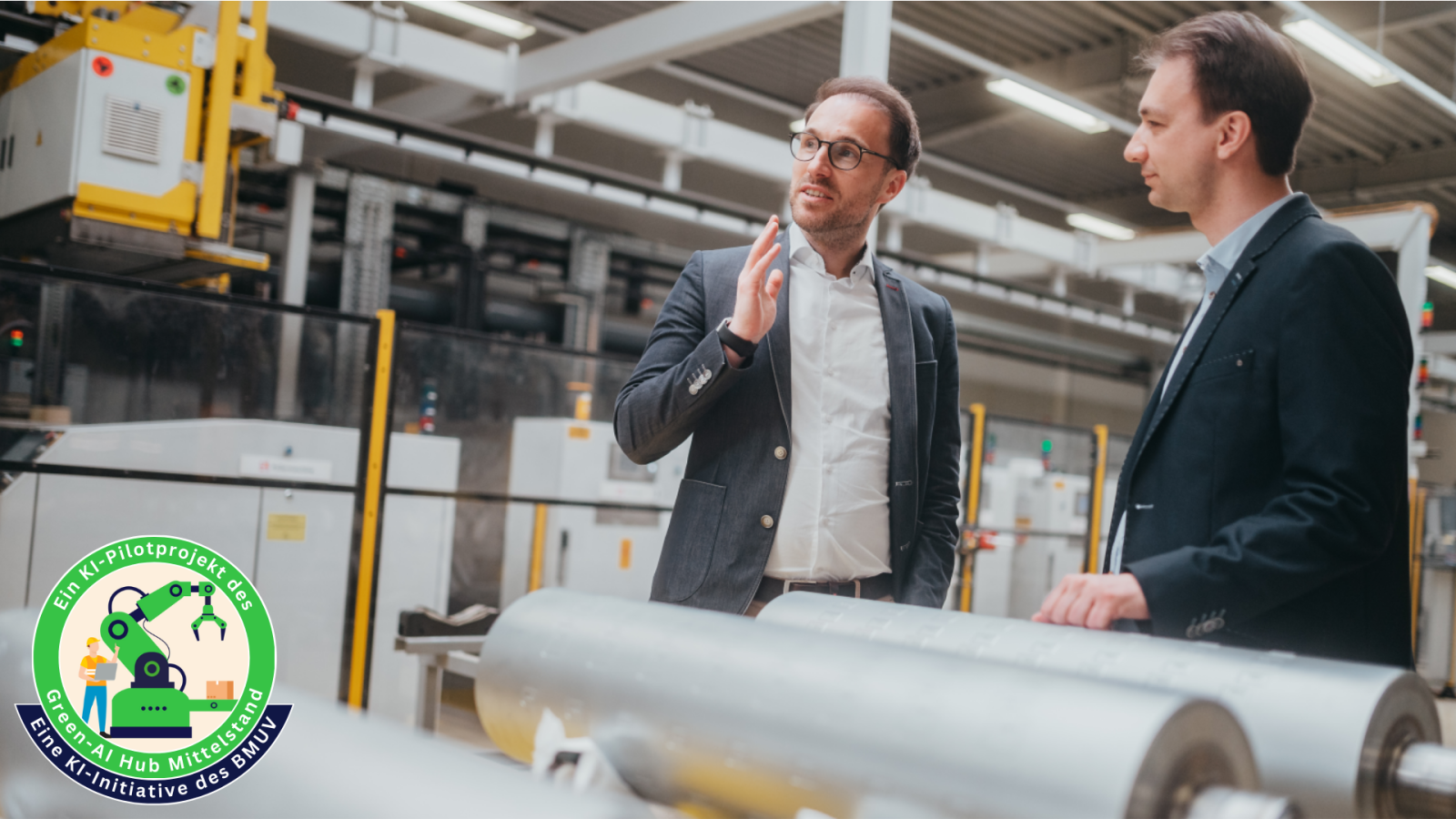
Innovative rotogravure cylinders production made in Teutoburg Forest
Founded in 2000, 4Packaging GmbH is a family-owned business located in Dissen at the Teutoburg Forest, Lower Saxony, near Osnabrück. The company employs about 120 employees who handle pre-printing processes such as artwork and reproduction, 3D packaging development, and the production of embossing and rotogravure forms. Their gravure form production process is notable for its material and energy use, along with a commitment to maintaining high quality.
4Packaging recycles the rotogravure cylinders used in gravure form production. This is comparable to a sheet of paper and a pencil, whereby the sheet of paper is always reused, and a new inscription is made possible by an eraser. In practice, this looks different: this involves depositing and applying micron-thin layers of chromium and copper on the cylinder. The copper layer is engraved with the print image, while the chromium layer enhances durability, allowing for extended use. These processes are energy-intensive and require chemical solutions for the galvanizing process. Many small parameters, such as the machine settings and the quality of the rotogravure cylinders, can influence the quality of the rotogravure cylinders and therefore the print result.
Adapting to technological changes and customer demands, 4Packaging continuously innovates its processes. 4Packaging pioneered the installation of an automated production line and has since expanded and modernized it. Their digital 3D packaging development allows customers to realize their ideas without the need for physical resources.
Challenge: improving process quality in electroplating
Maintaining reliable process quality is crucial in producing rotogravure cylinders. Even the smallest errors, for example during the so-called degreasing process, can lead to poor-quality rotogravure cylinders that are unusable in the subsequent printing process.
The quality of the rotogravure cylinder is also heavily influenced by the electroplating process. Electroplating is an electrochemical process for applying fine metal layers to a metallic or other conductive surfaces, such as copper and chrome. Various parameters determine the quality of the coating, including the pH value, current density, bath temperature, duration, or even the degree of contamination of the bath. The chemical baths are currently monitored manually by sending samples of the baths to the laboratory for analysis.
The Green-AI Hub Mittelstand pilot project introduces additional sensors to continuously monitor chemical baths and detect sources of interference early, significantly improving electroplating process quality.
Automation and optimisation of quality assurance through artificial intelligence
Artificial intelligence helps 4Packaging better understand the influences of the various process parameters on quality, and continuously determines product quality through visual image and machine data.
Artificial neural networks (ANNs) are used to analyse sensor data. This sensor data comes from two sources: the original sensors on the machines and systems, as well as additional sensors that were installed in the chemical baths as part of the Green-AI Hub pilot project, a process known as retrofitting. The neural network is used to determine the quality of the rotogravure cylinder. The AI is supplemented using computer vision (image processing), which automatically examines images of the rotogravure cylinders for defects and classifies the type of defect.
Implementing these technologies requires large amounts of data as well as labels, i.e. the categories that are assigned to a data set. For example, the computer vision model requires not only the image data, but also a marker indicating which defects are located were. The preparation of the data set for the computer vision model is initially a manual process where the markings are made by a person. Once an initial computer vision model has been trained, this data preparation step can be automated by the existing model, so that only a confirmation and a possible correction of an incorrectly interpreted error detection takes place.
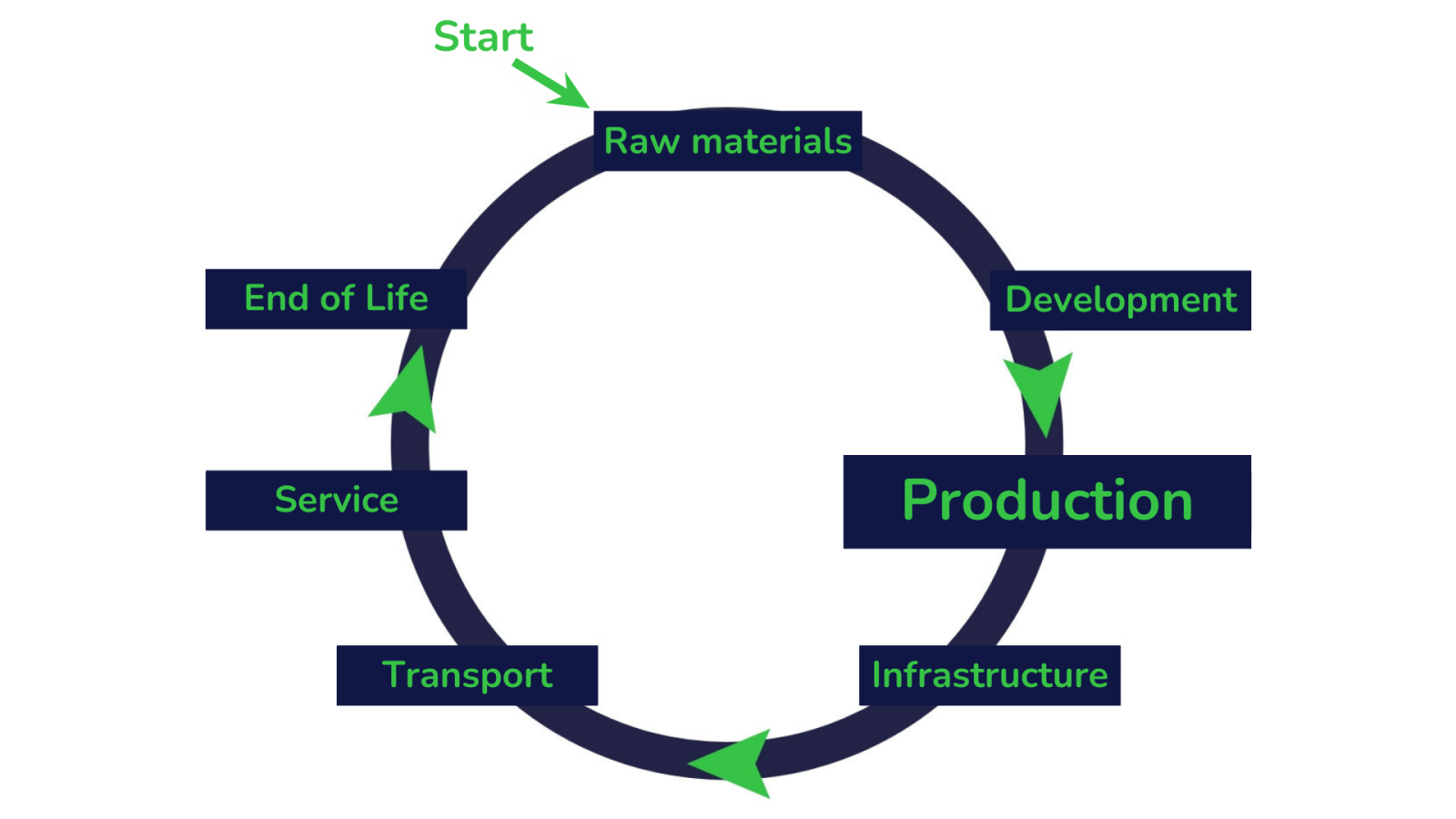
Saving resources through AI-based quality assurance
AI-based quality assurance at 4Packaging will automate manual quality checks so that deviations in the process are detected earlier. By improving processes with the help of AI-based quality assurance, 4Packaging expects to reduce defective rotogravure cylinders in the production process by 20 percent in the medium term, which would be up to 403 cylinders per year.
Potential savings can be achieved in terms of both the carbon footprint and the material footprint, which quantifies the lifecycle-wide material expenditure. By using computer vision and ANNs to analyse sensor data, the carbon footprint could be reduced by 9-12t CO₂ equivalents and the material footprint by 26-73t CO₂ equivalents annually.
Presentation of the Green-AI Hub pilot project “AI-based quality determination for rotogravure cylinders” - 10:37 min.
- Henning Hacker, 4Packaging GmbH
- Lukas Schießer, German Research Center for Artificial Intelligence GmbH
Technology
AI capability: data analysis and image recognition
AI model: artificial neural networks (ANN) and computer vision
Value creation
Phase: production & quality control
Aim of AI: automation of manual processes for quality inspection
Resource efficiency
Carbon footprint savings: 9-12t CO₂ equivalents
Material footprint savings: 26-73t CO₂ equivalents
Medium-term reduction of the error rate in the production process by 20 percent